Improved Last-Mile Supply chain Analytics for Pharmacy Chain by Migrating to AWS
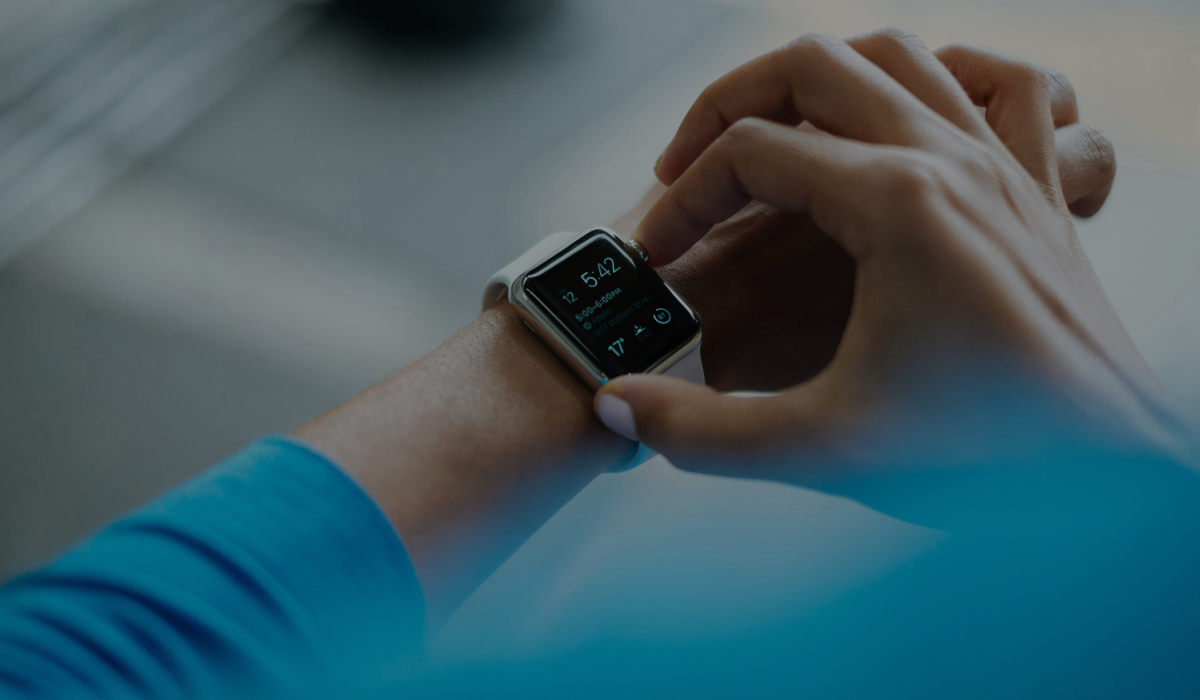
About Customer
Customer is a leading retail pharmacy and wellness products chain in India, operating a large omnichannel and hyperlocal retail network of 24x7 stores. The brand focuses on providing customers with a diverse range of pharmaceutical, nutraceutical, lifestyle, and wellness products with their catalog having over 1,00,000+ products, including curative & preventive medicines.
Business Problem/ Challenge
Our customer was using SQL Server and PowerBI for data visualisation, which resulted in high costs and limited analytical capabilities. They needed a comprehensive solution to consolidate all their data and support large-scale analytics. However, their existing setup was inadequate for handling extensive analytics workloads, including 1.5 TB of historical sales data and 1 GB of incremental data across over 250 tables. This hindered their ability to make informed decisions regarding sales and inventory.
Solution
To address the challenges faced by the customer, we implemented a comprehensive data warehouse solution.
The key steps in the solution included
- A robust ETL pipeline was established to handle the data extraction from different sources, transformation to ensure data consistency and quality, and loading into the data warehouse
- Implemented a scalable data warehouse solution using redshift, allowing for flexible and efficient storage and processing of large datasets. The data warehouse was designed to support complex analytics and reporting needs
- PowerBI is then pointed to the redshift which allows the customer to create dynamic reports and visualisations, enabling better insights into sales and inventory data
- Optimised the data processing workflows and queries to ensure minimal data processing time, significantly improving the speed and efficiency of analytics operations
Results and Benefits
- Seamlessly perform complex analytics and build dynamic reports and ML models across disparate data sources through data warehouse implementation
- Reduced data processing time from 6 hours to 30 minutes
- Improved decision-making by 80%